In this issue:
- Information Economies and Institution Economies—"Information economy" was a nice term for the positive side of service sector-driven growth, but it feels like a misnomer: we don't have many "information recessions," after all. Instead, a better description is that high-quality service-sector growth happens through better-quality institutions, and the harm of a recession is from breaking up some organizations and arrangements that worked well and are hard to rebuild.
- Distribution—Indirectly monetizing AI by using it to drive traffic to better-monetized non-AI products.
- Non-Tariff Barriers—The pettiest trade wars are survivable, just annoying.
- Low-Background Text—The cost of unique tokens.
- Application Layer—The newest software companies need to be valued the way they would have been in the 80s.
- Socks—It's hard to fight against a more flexible adversary.
This issue of The Diff is brought to you by our sponsor, Daloopa.
Information Economies and Institution Economies
For most of human history, up through roughly the 1960s, you could understand economies quite literally: they consisted of getting things out of the ground (plants, metals, energy sources), processing them in some way, and selling the outputs. Start with dirt, add labor and capital, and you get wealth. But starting in the 1960s, the richest economies hit peak manufacturing employment, which then started to decline—but they kept growing, both because manufacturing output per labor hour continued to rise and because of services.
It's a bit depressing to think of an economy driven by what a big portion of service sector employment is: waiting tables, ringing up customers, treating sick people, etc. These are obviously important tasks, but nobody dreams of an economy where most output is consumed at hospitals.[1] "Service economy" just sounds odd, and isn't especially inspiring, but starting in the 90s it was overtaken by the term "information economy," which just sounds a lot better. The idea was that, yes, shoe factories would move from the US to China. But Americans would still have jobs designing shoes, shooting Nike ads, shooting baskets in Nike shoes, etc. And all of these were higher-margin activities. Nike itself was basically a service economy company, with all that high value-added labor done in-house while the actual stitching, glueing, packing, etc. was done by companies with names like Fulgent Sun International Holding Co. Ltd.
There is a sense in which this is true: we do produce and consume far more information than we used to, and it keeps rising. Purely in terms of hard drive space needed to store the data, we're all producing more data in a few minutes of interacting with some apps than a good private investigator would be able to pick up in a day of following someone around. (That's true either if we maximally compress everything into text descriptions or if we count the actual amount of data—photo-sharing apps that will cache an upload of recently-taken photos are grabbing a few megabytes of data every time you interact with them after having taken a photo.) And a lot more economic activity is data-driven than before, partly from taking techniques that worked in the analog world and scaling them up—direct mail advertisers have been varying copy and coupons in order to see which offer drives more revenue for a century (Claude Hopkins was writing about it in 1923), and when late-night infomercials told you that "operators are standing by," they'd often vary the 800 number those operators answered in order to measure every detail of which parts of the ad kept viewers watching and which one got them buying. Doing the same thing in a few lines of Javascript is easier, and means that:
- Correspondingly more products and ads get continuously tested and improved,
- More of their value can be seen as the cumulative results of all of their previous experiments, and
- The techniques needed to analyze that information keep getting more sophisticated—an A/B test showing that "Buy Now!" does better than "Get Yours Today!" is easy to understand, but if you keep on testing, you're implicitly measuring the interactions between all of your previous tests—maybe "Get Yours Today!" is actually the optimal headline, but only if it's combined with a free trial and a higher price point. It's a combinatorial nightmare to keep all of these variables straight, and even harder when the underlying distribution changes.[2] (Sometimes a free trial is a great marketing hook when it's not the industry standard, but once enough competitors do it the customer base bifurcates into quality-indifferent users who cycle through trials and real buyers who don't, as happened with meal-kit companies and during the period where you could continuously borrow mattresses at 0% interest and sleep for free as long as you remembered to roll your Casper into a Nectar before the loan matured ($, WSJ).)
And it's suspicious that the Information Economy has never suffered a serious recession because hard drives got too expensive, or because someone dropped the wrong table. Hacks and IT snafus have hurt companies, but they're often correlated with other problems when they persist for a long time. And plenty of companies have recovered from them just fine: Reddit once had some of their users' unencrypted data stolen, and it wasn't a huge problem. (To be clear: Reddit was a very new, consumer-facing company, and it's probably socially optimal for companies not to heavily invest in information security at that stage. It was also a different, nerdier site, and the deep secrets users were sharing were things like "Lisp is cool, but I use Python at work" or "I don't 100% agree with Ron Paul." They're a much bigger organization today, with higher stakes, weirder secrets, and thankfully haven't had an issue like that in a while.)
Not only do we not have Information Recessions, but the US's last two recessions were actually bull markets in data: in 2008-9, with expanding margins—Google's EBITDA was 63% higher in 2009 than it had been in 2007, and social media grew through the downturn, too. And the brief Covid boom was also a wild bull market in data, as consumption shifted from harder-to-track in-person purchases to online ones, including online-mediated in-person transactions in the form of curbside pickup.
But there weren't many commentators talking about this as a driver of a rapid recovery, because it wasn't going to be one: there were too many countervailing negative forces. One of those forces was that while the economy was collecting and using more data in both periods, there were specific organizations that either had to rapidly reorient themselves or that went out of existence entirely. That's a necessary part of the economic process, and sometimes these shake-ups produce long-term benefits: companies hiring software engineers in 1999 couldn't afford to be picky, which meant that many organizations had very heterogeneous skill levels. But in the post-downturn period, the pickiest organizations were the most likely to survive, and they were doing all of the hiring, so they could have an incredibly high bar.[3] Early Google was lucky in that Microsoft was going through a bit of a tough time itself, with the DOJ antitrust verdicts, when Search was just beginning to take off in 2000. Microsoft faced a serious internal crisis of faith during this time period, which resulted in a notable exodus of talent.
Institutions create intangible capital because, over time, people learn to work well together, and they slowly select into organizations that run the way they like. If you read about truly odd cultures, like Valve's decentralized approach, Southwest Airlines' aggressive quirkiness, or Bridgewater's brutal honesty (all of these are exaggerated, but all seem directionally correct), you might find them off-putting, but some people hear about that and realize they've finally found a place that's just right for them. If those organizations shut down, human capital gets reshuffled inefficiently, and isn't quite as well-matched for the problems it's addressing.
Relationships between companies function the same way: Apple is really part of an Extended Apple that includes their entire supply chain and all of the sellers they work with. This has been built up over a long time, with OEMs designing their processes around what works for Apple and Apple designing its own timelines around what their suppliers can handle. When this works, it works incredibly well, and means that some sections of the free market are exceptionally well-planned mini-economies—but that their planning authority extends only as far as their ability to achieve roughly the results that they plan for.
So when recessions destroy wealth, and take a while to recover from, part of what they're doing is taking a carefully-ordered system and throwing a massive amount of entropy at it. The factories are still there, the people are still there, but the connections between them have to be carefully re-forged, and meanwhile the financial system, itself a complex network of specialized entities and complicated interrelations, is not good at injecting new risk capital when markets are seizing up and unemployment is rising. All that organization can over-optimize for the wrong situation: the US economy circa 1970 was extraordinarily well-designed as long as oil prices were low and stable, and the US in 2007 was a beautiful mechanism for achieving as much consumption as possible backed by the fact that local housing markets were uncorrelated and big banks only needed about a dollar of capital to back $30 of assets. When they're well-designed for not quite the right purpose, a negative shock can be worth it, and a counterfactual world where Lehman was rescued would probably just have shifted the financial crisis a few months forward in the future and a few hundreds of billions of dollars larger in magnitude. But even if they're necessary, they're still expensive.
Except to the extent that this is a super-abundant economy in which market forces have driven the cost of all other consumption close to zero, in which case health care spending is really a proxy for every other kind of spending: if merely existing means effectively unlimited access to utility-maximizing consumption, it makes economic sense for healthcare to be the biggest sector because the biggest bottleneck to enjoying all of that is just staying alive. Empirically, though, we find other ways to consume or even have a marginal savings rate approaching 100% as wealth compounds—there are many rich people who don't have to work, but only one Bryan Johnson. ↩︎
Multi-armed bandits and even more advanced variations like contextual and hierarchical bandits have been developed as a solution to these kinds of complex optimization problems, but the levels of complexity are a never ending march. ↩︎
For example, Victor Niederhoffer wrote that his father got a law degree in 1939, but couldn't find a legal job, but that the NYPD administered a standardized test to the 30,000 people who applied for their 300 open jobs, and since he scored in the top 100, he got hired. When you look at long-term charts of crime rates, consider how great the average cop was when unemployment was in the teens and the job paid well and had great job security. Early Microsoft was lucky in that one of the biggest US employers of smart engineers, Boeing, had been going through a difficult adjustment as it lost revenue from the Vietnam war and the space program, so they, too, were able to recruit plenty of talent. ↩︎
You're on the free list for The Diff. Last week, paying subscribers read about why markets being excessively responsive to news during highly uncertain periods is actually an efficient market in liquidity ($), why it takes more than four years to lose reserve currency status ($), and why white-collar jobs may follow the blue-collar pattern in the next recovery ($). Upgrade today for more upbeat coverage!
A Word From Our Sponsors
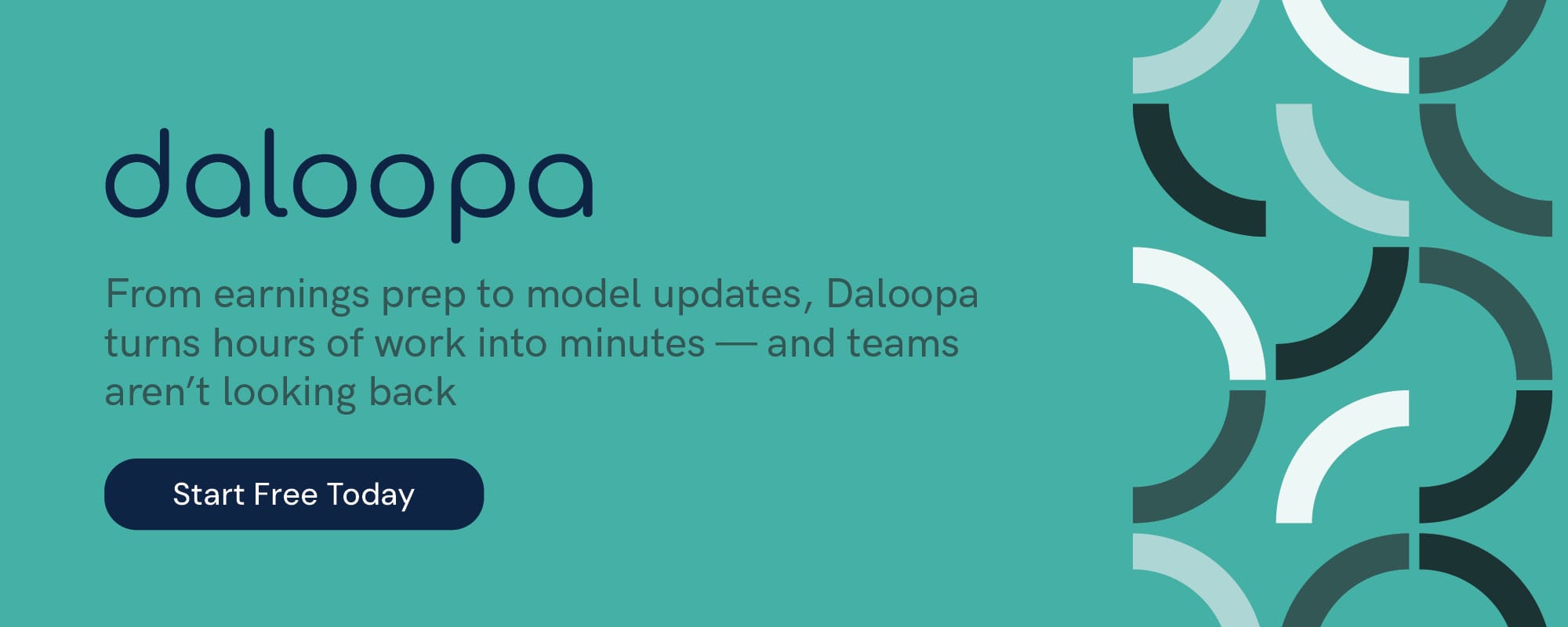
The funds moving fastest in this market aren’t copy/pasting numbers—they’re clicking.
Top hedge funds have shifted how they build and update models, source financials, and prepare for earnings calls. They’re using Daloopa to cut hours and days of manual work into seconds and minutes — and they’re not looking back. If you're still building and updating models from Qs and Ks by hand, you're already behind.
If your team is triple-checking numbers, they’re losing precious time. And if you're not using Daloopa, your competitors are gaining that edge.
Daloopa gives you 1000s of audit-ready, hard-to-get fundamental historical data to either build models or automate model updates all in one click. No more wasted time. Just faster, smarter work so you can do your job even better. The smartest teams are already in. Don’t get left behind.
Start your free account now and see why everyone’s switching to Daloopa.
Elsewhere
Distribution
Google is linking to its own search results within AI overviews. One of the qualitative differences between interacting with LLM chatbots and traditional search is that the point of search is to go somewhere else, but sometimes the point of using an LLM is to dive deeper down the same rabbithole. This presents a fun metrics challenge: one of Google's early advantages—not the biggest, but notable—is that it inverted one of its competitors' metrics: Yahoo wanted people to spend as much time as possible on Yahoo, so they'd see lots of banner ads, but Google wanted users to spend as little time as possible on Google, since that was a proxy for finding exactly what they needed. Today, close to 70% of queries don’t lead to clicks to open web properties.
Even before AI, Google had been evolving towards more of a destination. At a holding company level, they own one of the world's most addictive continuous-engagement platforms in the form of YouTube, but even in search, it's better for their product and much better for their monetization if more of the customer journey that starts on Google can stay there. AI is one more way to offer this, and inserting links to standard search results in the generative ones is a good way to steer a low-monetization query towards a higher one, and to desensitize users to the inevitable monetization of LLM results through ads.
Non-Tariff Barriers
The original Trump tariffs tried to target both explicit tariffs and implicit barriers to trade, using trade surpluses as a proxy for both. This was always a little bit odd: did Guyana start imposing some tricky new quasi-tariffs in the last few years, or is their trade surplus more a function of the country's GDP per capita rising over ~20x in the last twenty years because they discovered oil?
Even though that's a rough proxy, it's a good metric, and countries can and do limit trade without formal tariffs. China has been doing more of this to US products recently. Fortunately for long-term economic stability, this is more of an inconvenience than a catastrophe in many cases: if they make it hard for US companies to sell them LNG and beef, those companies will sell their products to countries whose previous exporters will be shipping to China instead. The net effect is still harmful, both to the global economy in general and to those industries in particular, but mostly because it means more goods will be in transit and there needs to be more shipping capacity to carry them.
Low-Background Text
A startup has reached out to at least one developer, offering to buy access to old, unused GitHub repos, ideally with pre-2022 source code. Open-source projects are such a great source of code paired with documentation that they're an incredibly obvious thing to include in a model that's intended to write code (or even one that's intended to reason about other topics—there's nothing like formalizing a question in code to make you realize you didn't quite ask the right one). Labs can and do hire software engineers purely to write code that helps train their models, but a nice feature of software is that it has a low marginal cost (at least, as long as it's not inference), so one way to get a unique-token windfall is to buy one.
Application Layer
AI companies are some of the fastest-scaling software businesses ever ($, FT), but the space is also notorious for businesses peaking early and then reversing course. One way to look at this is that it's actually a return to an older financial model for software companies: the generation that got big in the 70s and 80s was typically profitable from the beginning, because they made their revenue upfront; the idea of a software company continuously losing money for years while it slowly acquired customers was really a result of the rise of recurring revenue, which made the economics in any given year look misleading. But the faster a sector changes, the less it makes sense to talk about annual recurring revenue: the revenue-producing feature in question might be subsumed by a general-purpose model, or bundled by a different solution provider in the same space. The right response is probably to put a much lower multiple on these companies than their growth would otherwise justify.
Socks
US buyers have been pulling back from purchasing low-priced Chinese manufactured goods, like socks ($, WSJ). In one sense, that's perfectly fine: socks are very cheap, and plenty of countries are able to make them. But as China's pure cost advantage eroded, the country's supply chain developed other competitive advantages, like speed. So China's in a better position to quickly adjust to a new trade environment than the US.
Diff Jobs
Companies in the Diff network are actively looking for talent. See a sampling of current open roles below:
- A Khosla Ventures-backed startup that’s making homeownership affordable and feasible again by bringing assumable mortgages into the mainstream is looking for a product engineer with a strong track-record building complex products and systems. Experience with geographic, or real estate data is a big plus.
- A multi-stage, fintech focused investment firm is looking for an investment associate to support thematic opportunity identification, diligence, and execution. Investing experience OR high-growth operating/investment banking/consulting experience and demonstrated interest in fintech required.
- Well-funded, fast-moving team is looking for a full-stack engineer to help build the best AI powered video editor for marketers. Tackle advanced media pipelines, LLM applications, and more. TypeScript/React expertise required. (Austin, Remote)
- A premier proprietary trading firm is looking for smart generalists to join their investor relations team, working with external investors, rating agencies, and the internal finance team. Investment banking and/or investor relations experience preferred. Quantitative background and technical aptitude a plus. (NYC)
- A well-established asset-backed stablecoin project is looking for an analytical performance marketer to help scale their newly launched decentralized-ETF product and drive 100% MoM growth in total value locked. Experience managing sizable paid ads budgets and driving measurable growth required. (SF, NYC)
Even if you don't see an exact match for your skills and interests right now, we're happy to talk early so we can let you know if a good opportunity comes up.
If you’re at a company that's looking for talent, we should talk! Diff Jobs works with companies across fintech, hard tech, consumer software, enterprise software, and other areas—any company where finding unusually effective people is a top priority.