In this issue:
- Thinking-Things-Through Privilege—The more expensive it is to reproduce information, the better a strategy it is to instantly believe everything you read. But when information is close to free to generate and share, you need a different set of heuristics.
- Trade Negotiations—How long does it take to strike a trade deal?
- M&A—What the acquisition process looks like.
- AI and IP—Cheap signals of trustworthiness are most appealing to the least trustworthy.
- Consolidation—Waiting for the starting gun.
- Sourcing—Laggy supply chains as a competitive advantage.
This issue of The Diff is brought to you by our sponsor, Daloopa.
Thinking-Things-Through Privilege
Last month, the New York Times ran a fascinating story claiming that American homes are getting younger. If you read the story today, it says that American homes are getting older, because in the last ten years the average year of construction rose four years. This was not entirely their fault: the source is a report whose URL slug was us-median-home-age-trending-down/
, which now redirects to a new URL, us-median-year-built-on-the-rise/
. And the data points in the article make sense.
But the broader question is: how many people reviewed this and didn't check the calculation, or ask themselves what it would mean if the average age of housing stock declined nationwide. The article has an absolutely fascinating table of which locations have seen the fastest change in the median age of housing stock. It's a mix of oil and gas boomtowns (Williston, ND), extremely high-TFR religious enclaves (Monsey, NY; Lakewood, NJ), sun belt exurbs (Fort Mill, SC; Greenville, SC; Gallatin, TN), college towns (College Park, MD; Ithaca, NY; University Park, TX) and some recently-gentrified older cities (tear down an old building in Jersey City and replace it with a new high-rise and you can significantly decrease the average age of the housing stock even if population doesn't rise very much). So it really captures two phenomena: general growth, and a pattern of density, followed by urban blight, and subsequentgentrification. It also captures some noise, from very small metro areas where a single apartment building could swing the numbers, and presumably there will be a different mix if the snapshot captures a different one-decade range. All of this adds some texture to the issue, and spins off some other fun follow-up questions.^[Like: does this mean that cities with high economic growth create more consumer surplus in housing, since someone who has a preference for an old house with character or a new one with amenities can trade off between these desires while still staying within commuting distance? Does this mean that such cities have more aesthetic preference diversity? And does that mean that the city has less of a coherent identity? All fun things to consider!
All of this illustrates an important, growing distinction in cultural norms: in a world with an unlimited supply of content and data, you can produce coherent-sounding prose without thinking things through. It's a useful skill in some contexts, like talking about most company mission statements and most political platforms—in both cases, there's usually some amalgamation of principle and opportunistic compromise, but cast entirely as principle.[1] In an information-scarce environment, this approach will mostly mean repeating beliefs that have undergone either individual or group selection—there's individual selection for aphorisms, where a society that believes in "a penny saved, a penny earned" is likely to accumulate more wealth than one that doesn't, and where even factually-challenged beliefs that clearly delineate an ingroup and outgroup serve a coordinating function.[2] But in an information-abundant world, you can find a reasonably coherent version of any belief system, and you can probably also find a Discord server full of people who treat it as the truth.
There are some subcultures that vigorously preserve the norm of treating everything as up for debate. I'm not a rationalist, but am rationalist-adjacent in the very literal sense that at social events I'm statistically very likely to end up in close proximity to someone who's a member of a polycule, plans to have their head frozen when they die, donates a surprising amount of money to shrimp welfare-related causes, has donated a kidney, etc. They're a high-signal bunch, and one of their meta-norms is to try not to get offended by object-level upsetting ideas, which is incredibly refreshing. This norm does lead to an interesting dynamic, which is that a lot of the weirdest rationalist ideas come from laying out a few intuitive principles and then figuring out where they go, which means that rationalist debate feels like an ambush where you accidentally acceded to a few basic claims ("Sure, I guess animals feel pain. And yeah, if a puppy were in my way it would be nicer to gently redirect it than to drop-kick it") and then find yourself having accidentally laid out the first-principles argument for being a vegan.[3]
Some parts of capital markets and tech have this norm, too, but often in a more constrained form: there's a lot more risk-adjusted money in optimizing an existing process than in building a new one, and one part of optimization is having a nose for which projects will require high upfront effort before there's even any information on what their return will look like. It's hard to know what the optimum is: a company that never rethinks its business model will eventually die, because technology comes for every business eventually.[4] But a company that blows up its entire business model every few years is probably going to skip right past the optimum. The best compromise is probably to have a natural cycle of birth and decay within the business, and to continuously invent new business lines that are ideally complementary to the old ones, but that also have the potential to be riding up the steepest part of the S-curve when the legacy business begins to slow. (Sometimes it even loops around: Google's first source of revenue was powering search for other companies, and right now their fastest-growing segment is their cloud business, one of whose advantages is better search for their internal data.)
One of the better ways to stay in reasoning-mode rather than the default is to get your ideas from text rather than video. There's something about the moving image that's incredibly persuasive to people, and that also seems to short-circuit questions like "is this real? Why am I seeing this?"[^This is getting harder as even text (or image) native platforms are becoming increasingly video-mediated. One example is Facebook, where the percentage of total time spent watching video on the platform is up from 10% in 2015 to almost 55% today.] This problem predates generative AI, and arguably the ability to cheaply create a clip of a penguin trade delegation visiting Donald Trump innoculates people a bit. But one of the natural reasoning steps that's easy to skip with video is: who was filming this, and why? This is a point The Diff made years ago: a video of some kind of violent encounter often starts in the middle of it, or at least somewhere between when voices get raised and when fists start flying. You wouldn't make a video of a stranger on the subway asking the other to move a backpack off a seat, but you might start filming if one of them pulls out a knife. But if there's a conflict, it implies that each side thinks they're very much in the right (and will probably win). The viewer has to backfill the narrative that led up to it, and they're going to do that based on their preconceptions: "Why is that cop tasing that guy?" is a question you don't always get an answer to from the clip but that your assumptions about the trustworthiness of cops will happily supply to you. And, of course, the things that go most viral are the ones where responses naturally split 50/50, not the ones that one side doesn't even bother defending.[5]
Text doesn't perfectly protect you, either, of course. There have been high-impact books about fiscal policy that accidentally omitted some numbers from their sample that completely change the argument, and high-profile books about inequality that toggle between different measures of inequality to select the ones with the highest rates of inequality and which also mostly measured capital accumulation in terms of rising housing costs.[6] But text does have the advantage that all of us have similar production values, and that search, link roundups, social media, and email can still make text viral—especially in communities that prize contrarian arguments against high-profile books!
Expect this problem to get much worse as thinking gets easier to outsource. You can and should use LLMs as a way to refine arguments, identify examples, etc. But getting in the habit of doing that increases the utility of having good judgment about which beliefs and outputs to question. An LLM makes you smarter when you run your own writing through it and ask for weaknesses, but it makes you dumber if you just run every argument you disagree with through the same process instead. Models have reached a level of sophistication, and their moderation has been hobbled enough that they can come up with a cogent case for or against any proposition you can think of, if that's what you ask them to do. And that means that having the habit of asking the right questions, even when it's uncomfortable, is the only hope for being right.
This is especially easy to see when the parties flip positions on some issue, like trade, but keep their other positions. It's just not the case that someone sat down and thought really hard about what their views on abortion, gun control, and capital gains taxes imply about trade barriers and concluded that they'd gotten things backwards. Instead, a very successful coalition entrepreneur implicitly realized that he could mobilize older, less educated voters with a more protectionist message. Clinton was able to achieve something similar by making his party more of a college graduate-oriented coalition, and Reagan was good at uniting libertarian economic views with rising religious conservatism. On the corporate side, Google's mission is "Organize the world's information and make it universally accessible and useful." But there are some subsets of The World's Information that they are very reluctant to organize, such as information on suicide methods or data on what people look like when they aren't wearing any clothes. There are great business reasons to do this, and principled ones, too, but they don't fit into a mission statement unless it's going to be book-length plus appendices on special cases, or something terse and Orwellian like "Organize the world's information, remove all the bad kinds, and make the rest universally accessible and useful. Also, don't tell anybody that this is the real policy, lest they decide to see what secret naughty things they can find on Bing." ↩︎
For example, chauvinism raises the friction of dealing with the outgroup, but lowers it for dealing with the ingroup. And members of the ingroup are easier to reputationally punish if the dealings go badly. So there's an optimal level of bigotry that falls as a function of how effective the state is at enforcing contracts—one reason so many of the world's legal codes are descended from those of large ethnically and religiously diverse empires. ↩︎
For the curious, I lean towards deontology over utilitarianism for roughly the same reason that rationalists, at their day jobs, import libraries and use API calls rather than constructing their own programming language and implementing everything from scratch—there are some domains that naturally abound with edge cases and paradoxical issues, and while it's incredibly gratifying to explore these, it's also important to actually get stuff done—and the doing-things process also produces information that mere theorizing won't. Solving morality is premature optimization, and finding some preexisting social structure that allows you to be, say, 85% compliant with a system that's 85% true is probably a better bet than getting to 99% compliance with something whose truth value takes a few generations to determine. ↩︎
Even the durable ones! There are universities, hotels, and banks that have been in almost continuous operation for centuries, but they all needed to adopt websites, electricity, and indoor plumbing, etc. ↩︎
One thing that's changed since that essay first came out is that defending the most deplorable things your side has done has become more of a default winning move in political circles. But sometimes you can tell that their heart isn't really in it or that they represent a fringe viewpoint within the side they're on. ↩︎
If higher housing costs drive a significant share of inequality, it's still a policy problem, but one that you solve at the level of a few high-rent cities rather than at the level of income tax rates, at least if you're trying to minimize deadweight loss. Demographic factors can also affect this: if people work longer, and if the value of their work compounds in the form of dense personal networks, then some of that value will be reflected in high real estate prices. But that remains a Georgist critique rather than an argument for social democracy. ↩︎
You're on the free list for The Diff. Last week, paying subscribers read about how endless ambient entertainment changes the market for low-skill labor ($), why some AI companies are gigs, not institutions ($), and how to think about the fundamenatls of the VIX ($). Upgrade today for full access.
A Word From Our Sponsors
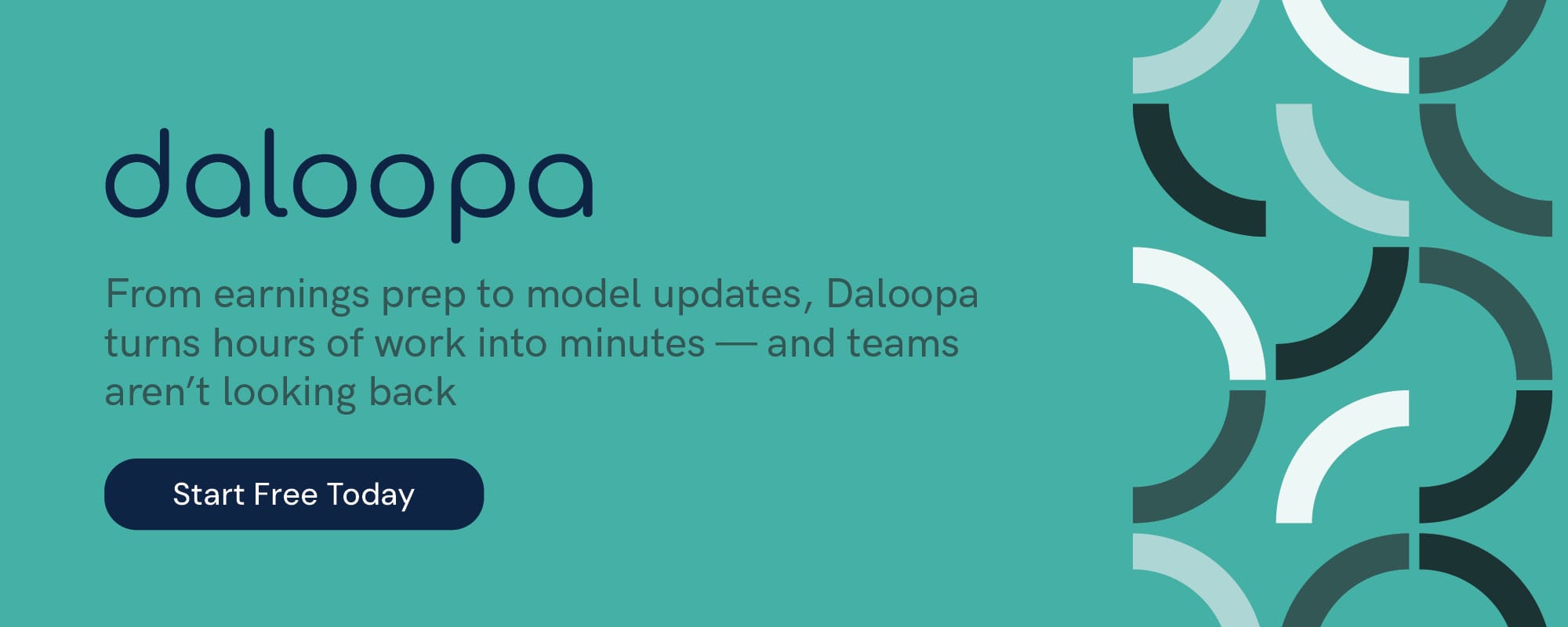
The funds moving fastest in this market aren’t copy/pasting numbers—they’re clicking.
Top hedge funds have shifted how they build and update models, source financials, and prepare for earnings calls. They’re using Daloopa to cut hours and days of manual work into seconds and minutes — and they’re not looking back. If you're still building and updating models from Qs and Ks by hand, you're already behind.
If your team is triple-checking numbers, they’re losing precious time. And if you're not using Daloopa, your competitors are gaining that edge.
Daloopa gives you 1000s of audit-ready, hard-to-get fundamental historical data to either build models or automate model updates all in one click. No more wasted time. Just faster, smarter work so you can do your job even better. The smartest teams are already in. Don’t get left behind.
Start your free account now and see why everyone’s switching to Daloopa.
Elsewhere
Trade Negotiations
Historically, it takes a year and a half, on average, for the US to negotiate a trade agreement, and almost four years to go from the start of negotiations to full implementation. They're always difficult, because trade policy is a domestic issue, and one with the classic problem that any given trade barrier has more concentrated immediate benefits and diffuse immediate costs. It is, for example, not a good policy for the US to protect its sugar industry, which would be viable albeit smaller with less protectionism. But it's great politics, because sugar's share of the average American's budget is around 0.2% (80 pounds consumed per year, ~$1/pound, versus a median income of ~$40k), while sugar's share of a sugar farmer's income can be 100%. Nobody is getting voted out of office purely because sugar protectionism costs the average American a trivial amount each year. And sugar seems to be a random category that got a bit lucky politically. You have to figure out what weird political dependencies other countries have, too, in order to know which products are hot-button issues and which are safe. Meanwhile, your negotiation counterparty has an incentive to mislead about this: the more industries they describe as political hot-button issues, or of special interest to powerful legislators, etc., the more of a concession it appears to be if they let that industry face a bit of competition.
So, these deals are hard to strike, except insofar as the deal is a return to the status quo. Even an effort to craft a deal that maximizes the perceived change while staying close to what already existed is a challenge—the original deal was already designed to be as politically popular as possible given its goals, so tweaks look bad. There's a claim that Japan and the US are close to a deal, though Jeff Stein at the Washington Post says that's not true. Whatever the trade deal is, unless there's some incredible efficiency unlock ("let's just give two AI agents equal tokens and let them negotiate until they reach an agreement") it won't look like the ones we're used to.
M&A
M&A deals underperform expectations notoriously enough that outlining how this happens is an entire academic subfield. One reason for this is that it's hard to forecast the future and very easy to fall prey to narrative bias. As it turns out, even the winning ones do. Meta's internal deliberations ahead of the Instagram acquisition are worth reading because some of the core points are right—Instagram was getting more sharing than Facebook, and even Facebook employees liked it—but they were also worried that Instagram was a threat if someone else bought them, like Google. Given the information they had, when Facebook bought Instagram it meant that Instagram had been scooped up by a $100bn company to keep it out of the clutches of a company worth $200bn. But Meta also underestimated how effective Instagram could be: their old model was to acquire companies, maintain their products, but move their teams to the core Facebook app. As it turns out, Instagram was worth buying as a standalone business, not just so it could be kept on life support while Facebook took over its use case.
Disclosure: long META.
AI and IP
Actors who licensed their likenesses to AI video companies are alarmed that they're the face of scams. It was always the case that AI ads would be better for direct response than brand ads—the lift from precise targeting is worth the hit from a hallucination in that context, but not when the ad is meant to be permanently associated in viewers' minds with the advertiser. What was less obvious is that one of these use cases is in running scam ads:
- At least some real actors will balk at jobs like being the spokesperson for an outright fraud, but
- Going to the trouble of producing a video does demonstrate some minimum financial investment in the outcome, which at least correlates with being on the level. As soon as the cost of some signal of trust declines, the people who exploit that the fastest are the ones least deserving of trust.
Consolidation
Last year, The Diff wrote a bit about Mario Draghi's proposals for improving European competitiveness, with a particular focus on telecom M&A as the most actionable proposal ($), since it involved deals that made economic sense and because all the government had to do to make this happen was credibly commit to not intervening in deals. That seems closer now, but apparently European telcos are waiting for someone else to strike the first deal so they can see what the regulatory landscape really looks like ($, FT). Mergers sometimes come in waves, because concerns about mergers being blocked are a form of potential energy, and the longer those concerns last, the more obvious deals there are that are waiting for a catalyst.
Sourcing
One of the annoying features of a complex economy is that growth is sometimes an invisible process of some things getting slightly better and other things, having gotten better, getting gradually more expensive as companies harvest the surplus they've created. The WSJ has a fun portrait of this in the form of a story about Home Depot's flowers business ($, WSJ): Home Depot's single largest revenue source is gardening, and as they've grown, they've gotten to a position where flower breeders compete in a centralized venue to get Home Depot's business, after which their plants get tested in a few dozen growing centers ("For security purposes, some of those experimental gardens are hidden in cornfields or through backyard donkey corrals, protected on secret farms before the plants are selected and patented"), and only then scaled up and sold. That adds a lot of lead time, but it also means that there's lead time for anyone who wants to catch up.
Diff Jobs
Companies in the Diff network are actively looking for talent. See a sampling of current open roles below:
- A Google Ventures-backed startup founded by SpaceX engineers that’s building data infrastructure and tooling for hardware companies is looking for a software engineering manager with 7+ years experience building large scale distributed systems. (LA, Hybrid)
- A multi-stage, fintech focused investment firm is looking for an investment associate to support thematic opportunity identification, diligence, and execution. Investing experience OR high-growth operating/investment banking/consulting experience and demonstrated interest in fintech required. (NYC, London)
- YC-backed company that’s turning body cam footage into complete police reports and automating other cumbersome law enforcement workflows with AI is looking for a junior backend engineer to operate in a forward deployed capacity. Entry-level ok, ability to pass an FBI background check required. (SF)
- YC-backed startup using AI to transform how companies quantify and optimize engineering productivity is hiring formidable full-stack and AI engineers. Experience with React + Typescript, Go, or Python on the ML side a plus. (SF)
- A hyper-growth startup (10x growth in 9 months) that’s turning customers’ sales and marketing data into revenue is looking for a head of deployments who is excited to work closely with customers to make the product work for them. Experience as a forward deployed engineer and leading enterprise deployments preferred. (NYC, SF)
Even if you don't see an exact match for your skills and interests right now, we're happy to talk early so we can let you know if a good opportunity comes up.
If you’re at a company that's looking for talent, we should talk! Diff Jobs works with companies across fintech, hard tech, consumer software, enterprise software, and other areas—any company where finding unusually effective people is a top priority.